Ethical AI - How Fama is combatting bias in AI with diversity
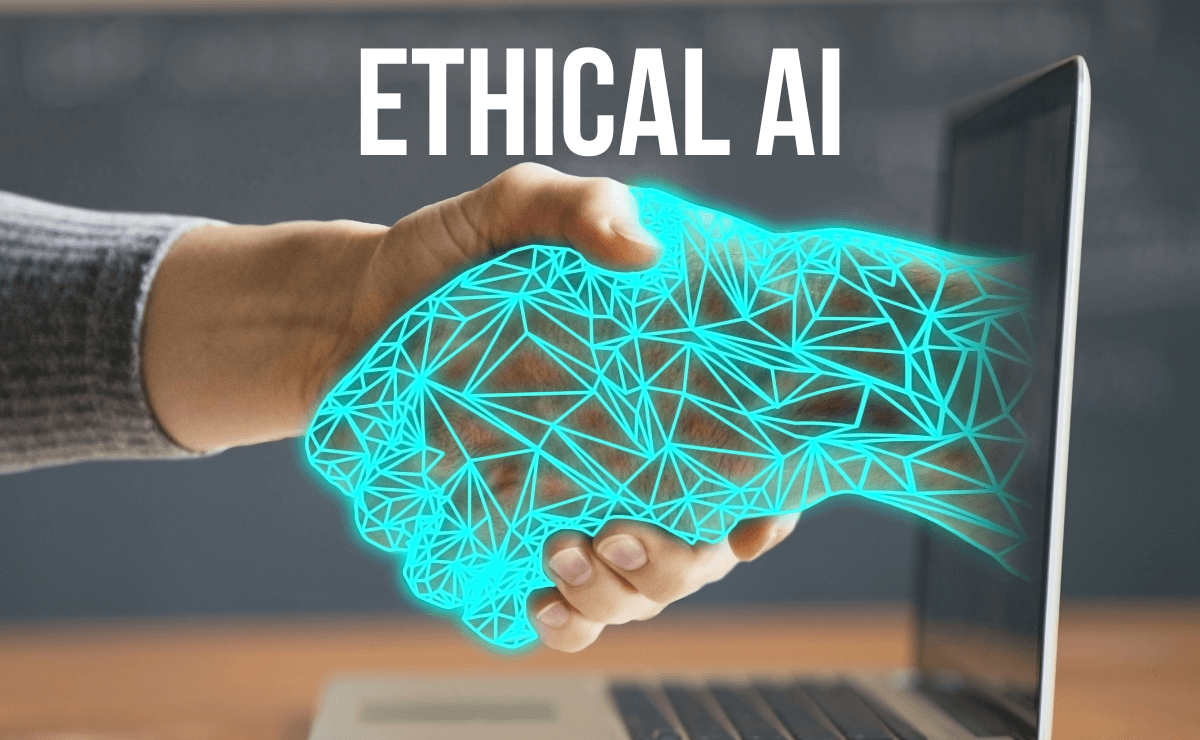
Welcome to our inaugural Fama Tech Blog! In this episode learn how Fama approaches AI from Fama CTO Brendten Eickstaedt.
Eliminating bias in AI and machine-learning technologies remains one of the biggest challenges facing the technology industry today. Between sexist hiring algorithms and racially biased facial recognition software, we have seen no shortage of discouraging headlines over the years that point to the growing need to ensure, from the earliest stages of development, that the tools we create aren’t reinforcing society’s most harmful prejudices.
For this episode of our Engineering Blog interview series, we sat down with Fama’s own Chief Technology Officer, Brendten Eickstaedt, to discuss this topic in more depth, and more specifically to explore the solution of combating bias in AI with diversity.
Here are a few key takeaways:
The Three Types of Bias in AI
In order to solve the problem of bias in AI and machine-learning technologies, we first need to understand exactly why it happens in the first place. According to Brendten, the artificial intelligence community currently identifies at least three distinct types of bias that may result from the use of AI: algorithmic prejudice, negative legacy bias, and underestimation.
Algorithmic prejudice occurs when otherwise legally protected features, such as race, can be revealed through correlation with other non-protected factors. For example, while zip codes aren’t considered protected attributes for the purpose of employment screening, they can be used as a proxy for race, inadvertently revealing the protected feature and potentially leading to a biased hiring decision.
Negative legacy bias, on the other hand, occurs when outdated information is being fed into an algorithm, which can easily result in an AI factoring in the toxic stereotypes of our less-than-sterling past, such as “traditional” gender roles.
Finally, underestimation is the result of an AI or machine-learning algorithm being trained on either insufficient or unbalanced data. If a company trains an AI to select candidates based on the attributes of existing employees, but the overwhelming majority of existing employees happen to be white males, then the AI is making decisions based on an objectively unbalanced data set that favors one demographic over another.
The Importance of Diversity of Thought
Despite all of the above biases seemingly taking place at the level of the algorithm, it’s important to remember that human beings are still ultimately responsible for both the initial development of the technology and the process of improving the AI’s performance over time. And for Brendten, this is one of the reasons it’s absolutely critical to leverage diverse teams in both the development of Fama’s screening algorithms and the analysis of their results.
“Team members with diverse backgrounds drive diversity of thought,” he says. “So it’s important to have those diverse teams to be able to think creatively and solve problems in ways that a non-diverse team would potentially have trouble with.”
To illustrate more clearly how diverse teams can help reduce or eliminate AI bias in practice, Brendten elaborated on Fama’s own process for improving its machine-learning algorithms. When an AI is returning incorrect or biased results, he explains, human intervention is necessary to retrain the algorithm not to repeat the mistake.
This retraining process is often referred to as a “feedback loop,” and consists of feeding new information into the algorithm that reconciles its previously biased point of view. Naturally, more diverse teams tend to produce more varied—and ultimately more balanced—information, and as Brendten puts it, “the key to keeping bias out of an algorithm is to be constantly retraining it on unbiased data.”
Importantly, Brendten is (thankfully) far from alone in this perspective. Other experts in the field have recently come to similar conclusions, regarding both the importance of the human component in future developments of the technology, as well as the notion that diverse teams are far more likely to build less biased, and ultimately more useful AI and machine-learning models going forward.
Should we be Afraid of AI?
While it’s safe to say the majority of the global population has abandoned fears about AI promoting an apocalyptic, Terminator-esque invasion of intelligent machines, there are many who remain discomforted by the much more realistic issue of AI bias. In fact, for some the aversion is strong enough to prompt the question of whether AI should even be allowed to exist.
In response to this point of view, Brendten reiterated the importance of remembering the human component. More specifically, he emphasized the critical point that AI and machine-learning technologies are merely tools that allow us to perform beyond our human limitations.
“It’s critical not to lose sight of the fact that AI and machine learning is designed to help us do things that our brains aren’t necessarily great at, which is processing data really fast,” he says. “But as humans, we also have the general ability to recognize patterns that AI can’t.” Moreover, Brendten again stressed the role of diversity in ensuring that as many negative or problematic patterns as possible are recognized by those responsible for developing and refining the technology.
As far as whether AI should exist, Brendten shares the view of Timnit Gebru, a leading AI ethics researcher and former Google developer, who believes that it’s ultimately the responsibility of AI developers to confront that question themselves, ideally before unleashing any new technology on society. In an interview with Vox, Gebru addressed recent concerns related to biased facial recognition technology, taking closer aim at how the technology would ultimately be used rather than how it functioned.
“It’s not about ‘this thing should recognize all people equally.’ That’s a secondary thing,” said Gebru. “The first thing is, what are we doing with this technology and should it even exist?”
At the end of the day, Brendten too believes that truly reflecting on this question should be a prerequisite for developing new iterations of AI. But perhaps even more important than the question itself, is ensuring the freedom from bias of the very people who are tasked with providing an answer.
Fama is the largest social media screening company and a leader in applying AI to background screening services. With Fama, organizations can proactively protect their culture and brand. Having raised over $27M Fama is headquartered in Los Angeles, CA, with employees all over the globe.
We invite you to watch the interview with Brendten below and join in on the conversation.
Get the Newsletter
Recent Blog Posts

The Future of AI: From Room-Sized Machines to Pocket-Sized Supercomputers
