The EU Fama and the Use of Algorithmic Decision Making
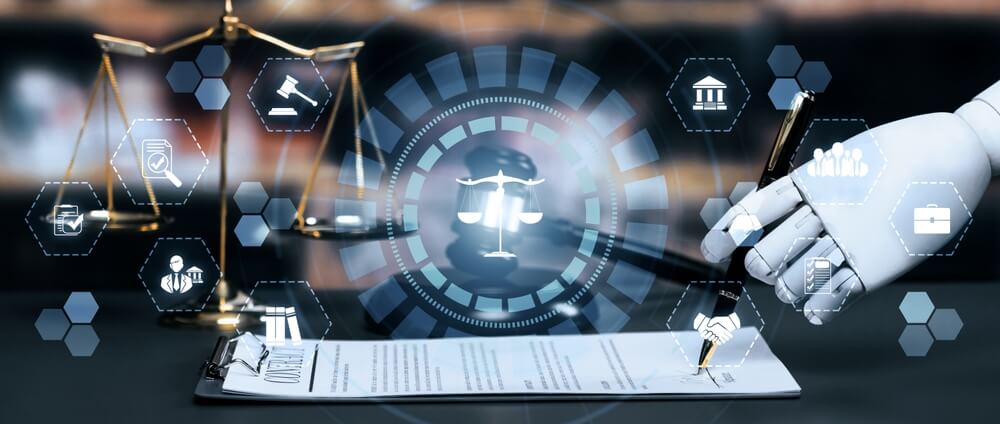
We are at an inflection point with the concept of algorithmic decision making that has elevated awareness of the inherent risks such processing poses. Interestingly enough, the risks AI poses are, at their core, very similar to the risks that human decision making poses. The primary difference is that human decision making has over a thousand years of trial and error in figuring out how to address its risk of harm, at least in the landscape of Western culture. This experience is not present with AI.
This paper is designed to advance the conversation by examining how the EU and others have taken steps to reduce the risk of such harm via legislation and policy, with a particular focus on GDPR and the AI Act. We also aim to describe how Fama has enrolled within this framework, and staged a successful international expansion.
âGeneral Considerations Related to Algorithmic Decision MakingÂ
Letâs start at the beginning.Â
The use of algorithmic decision making has been around for some time now. Additionally, with any powerful tool or technology, any possible potential for good is coupled with an equally powerful potential for âbad.â The risks associated with this kind of activity have been perceived long enough that both regulation and best practices have evolved to address the potential pitfalls associated with algorithmic decision making. Now, in the 21st century, the advances in artificial intelligence (âAIâ) reflect the need to ensure that the risks related to algorithmic decision making continue to be effectively mitigated. While not truly new, modern AI applications have a geometrically larger potential for both good and bad outcomes as it relates to individual rights and protections.
Despite not using the term âartificial intelligenceâ per se, there are a number of laws and regulations which impact the use of AI. These requirements span all levels of government. The EUâs General Data Protection Regulation (âGDPRâ) provides individuals the right to âopt-out,â or object to âsolely automated processing.â The California Consumer Privacy Actâs regulations are directed to set out mechanisms to permit a similar right to opt-out of automated decision making and âprofiling.â New York City has passed a local ordinance which requires bias audits before any AI can be deployed in an employment context.

There are also efforts to tackle the concept of AI directly. Starting in 2021, the EU approach is the Artificial Intelligence Act. The UK is taking a less âlegislativeâ approach with a policy paper on AI uses. The US White House has taken a bit of a middle ground approach in 2022 with an AI âbill of rightsâ policy paper, but requires Federal agencies to study how AI is used within the agencies (and the risks associated with such uses). As the speed with which AI is deployed âin the wildâ increases, regulation also increases.Â
Fundamentally, the risks of algorithmic decision making are the same as the risks that a number of laws are designed to address â even in the human decision making context. Bias, incorrect data driving decisions, and irrelevant data causing adverse impacts on individuals are all very real risks. In point of fact, GDPR is one of those laws noted above that implicates algorithmic decisions without calling it AI. Consequently, it follows that any development or deployment of AI needs to address these kinds of risks, as well.
âEU Law IssuesÂ
With GDPR and the AI Act taking center stage in addressing the concepts of risk in algorithmic decision making, the question then becomes âwhat do we have to do under these laws?â As the AI Act is not yet final, we will turn our attention to GDPR.
GDPR starts off with some baseline requirements related to ANY processing of personal data, and these requirements are present for algorithmic decision-making, as well. Note that since these are material limitations on processing, the AI-facing article of the GDPR (i.e. Article 22) is âin addition toâ and not âseparate fromâ the baseline requirements.â
Initial Requirements â Processing Principles & âLegal BasisâÂ
For any implementation of algorithmic decision making, the processing has to follow Article 5 in terms of the underlying principles, and the processing has to fit within one of the âlegal basesâ for processing under Article 6. Consequently, any algorithmic decision making has to process data, which is:
- processed lawfully, fairly and in a transparent manner;
- collected for specified, explicit and legitimate purposes and not further processed in a manner that is incompatible with those purposes (with some enumerated exceptions that generally donât apply in the context of the use cases discussed here);
- adequate, relevant, and necessary in relation to the purposes for which they are processed;
- accurate and, where necessary, kept up to date;Â
- stored for no longer than is necessary for the purposes for which the personal data are processed (also with some enumerated exceptions); and
- maintained in a way that ensures appropriate security, including protection against unauthorized processing, accidental loss, destruction, or damage.
â
Each of these principles has to be followed for any processing of personal data, and especially for algorithmic decision making. However, even if we follow the above six principles, there still needs to be a âlegal basisâ for such processing. This is a result of the first principle noted above. However, the EU has a different view as to what constitutes a âlegal basis.â In the US, unless processing of data is actively prohibited, such processing is âlawful.â In the EU, this is not the case. In order for personal data to be processed, such processing must be for one of six reasons (the âlegal basisâ for processing):
- the data subject has given consent to the processing (and there are rules around what constitutes âconsentâ);
- processing is necessary for the performance of a contract where the data subject is party (or in order to get the data subject to execute such a contract);
- processing is necessary for compliance with a controllerâs legal obligation;
- processing is necessary in order to protect the vital interests of the data subject or of another natural person (âvital interestâ here is an interest usually associated with health or safety);
- processing is necessary for the performance of a task carried out in the public interest (this requires the government to tell you to carry out the task â you just canât say that something is âin the public interest.â For example diversity isnât âin the public interestâ without some government mandate that it is); or
- processing is necessary for the purposes of the controllerâs (or potentially a third partyâs) legitimate interests, except where such interests are overridden by the data subjectâs interests. Note that the data subjectâs interest doesnât have to be âlegitimateâ like the controllerâs interest does.
â
How each legal basis can be used to justify algorithmic decision making is important since the different legal bases interact with each other differently. For example, if consent is used as a legal basis for processing, no other legal basis may be used. This is because for consent to be valid, it must be easily revocable. Thus, no âdaisy chainingâ of legal bases are allowed when consent is the legal basis used. The other five legal bases can be used concurrently. Additionally, not all the identified legal bases can be used as a basis for screening based on a personâs web presence. Generally, consent is the most reliable way to ensure the appropriate basis for such processing is covered.
Automated Processing and Profiling (Algorithmic Decision Making)
Once it is established that all the principles under Article 5 have been met, and there is an Article 6 basis for processing, we next move to the requirements around Article 22 â which are most applicable to algorithmic decision making.Â
Article 22 is generally framed in a negative voice. While Articles 5 and 6 are proactive (âthese are the things you must doâ) Article 22 is restrictive (âyou may not do these thingsâ). This is mostly due to the underlying policy position against automated decision making that is embedded in the GDPR. The primary function to Article 22 is to allow individuals to avoid having decisions made about them based on âsolely automated processing.â
Note that not all automated processing is subject to this general prohibition â only solely automated processing. As noted above, since human decision making has the experience that AI decision making doesnât, the use of such human decision making is an important check and balance on any algorithmic determinations. This fact is reflected in the GDPRâs treatment of automated decision making. Namely, if there is human involvement, Article 22âs general prohibition doesnât apply.Â
However, AI-based processing, and many deployments of algorithmic decision making do not have human intervention in the decision making process. As such, those should be considered âsolely automated processing.â It is worth noting that âdecisionsâ must have a âlegal effect,â or a âsimilarly significantâ effect. While this limitation may be perceived as narrowing the scope of Article 22, it is important to remember that there are many more âsignificantâ effects under EU law than under US law. As such, determining if algorithmic decision making can have a âsignificant effectâ is an important part of deploying such technologies.
There are exceptions to the blanket prohibition under Article 22. The most obvious is consent. However, as noted before, consent is a more difficult thing in the EU than it is in the US. Under EU law, consent has to be: 1) affirmative, 2) free, 3) informed, and 4) revocable. âImplied consentâ doesnât work in the EU. Thus, anyone who is subject to algorithmic decision making as a result of their consent has to be able to stop the use of the technology (at least as it relates to their data and its ongoing storage and use).
Besides using consent as a basis for solely automated decision making, such decision making can also be undertaken when it is either specifically authorized by EU or local law, or is required to enter into, or perform under, a contract with the data subject. For example, if German law specifically called out the need to use AI to evaluate the reliability of online content published by a âplatformâ (which seems to be the case in the EUâs Digital Services Act), then consent wouldnât be needed. Similarly, where solely automated processing is required to enter into a contract (as where a shopping cart engine evaluates the availability of a product in a warehouse before letting you pay for it), consent is also not needed.Â
Unfortunately, these non-consent bases for solely automated processing are very narrow and donât cover a wide enough range of activity to allow for algorithmic decision making in a screening context without consent.
The resultant effect is that either consent is needed, or automated processing will require human intervention in order to protect the individual rights and freedoms of individuals.
High Risk ProcessingÂ
Data Protection Impact AssessmentsÂ
Assuming that we have fulfilled all the principles of Article 5, have found a âlegal basisâ for processing under Article 6, and have gotten the consent (or used one of the other two exceptions) under Article 22, the deployment of algorithmic decision making may still not be permissible under the GDPR. This may be because such processing is viewed as âhigh risk.â
Whenever processing (in particular when it uses new technologies) is likely to result in a high risk of compromise of the rights and freedoms of individuals, a Data Protection Impact Assessment (âDPIAâ) needs to be undertaken. Considering the nature of algorithmic decision making, especially solely automated algorithmic decision making, it would be difficult to see how such processing wouldnât be considered âhigh risk.â However, the risk rating of algorithmic decision making can change depending on the mitigating controls applied to such processing. Consequently, while algorithmic decision making may have an âabsolute riskâ rating of âhigh risk,â where effective mitigating controls are implemented, a DPIA can show that the âresidual riskâ is moderate or low.â
Regulator InvolvementÂ
Assuming that a DPIA can show that the particular deployment of algorithmic decision making is not high risk, the inquiry should be able to stop there. However, it may not be possible to show that the specific deployment has sufficient mitigating controls to remove the processing from the âhigh riskâ category. In such an event, the specific deployment of algorithmic decision making may still be possible (though more difficult). GDPR Article 36 permits controllers to consult with competent supervisory authorities in order to get an âofficialâ determination that the âhigh riskâ processing is permissible.Â
Under this process, if the controller canât get a deployment of algorithmic decision making out from under the âhigh riskâ designation on its own, the controller may approach its supervisory authority to get help in determining what additional (or alternative) mitigating controls will reduce the risk of the processing to acceptable levels. This way, if the supervisory authority approves of the deployment and its mitigating controls, it will be much more difficult to have another supervisory authority, or even an impacted data subject, complain that the algorithmic decision making violates oneâs privacy rights.â
Trust in the System
Fundamentally, the use of AI or other algorithmic decision making systems need to have a demonstrable measure of trust by the stakeholders in the ecosystem â in this case the data subjects, the regulators, and the other market participants who use such technology. The GDPR does provide a pathway to generate this kind of trust. Compliance with Articles 5 & 6 are the beginning (as they are needed for any form of processing, not just algorithmic decision making).Â
Once those hurdles have been cleared, then there is the question of whether or not the algorithmic decision making is âsolely automated processing.â If it is, then there are ways to inject trust at that point (i.e. consent). If such processing has human interaction and direction in the decision making, then the strictures of Article 22 arenât needed.
And finally, even if there isnât âsolely automated processingâ going on in the decision making, the use of algorithmic decision making â even with human oversight â may still be considered âhigh risk.â As a result, a DPIA being undertaken should have the result of showing the mitigating controls which take an inherently âhigh riskâ processing activity and reduce the risk to acceptable levels. While it might take the cooperation of the regulators to get there, having a documented model of controls that reduces risk should elevate trust in a system that deploys algorithmic decision making.
âFamaâs Approach to Algorithmic Decision Making in ScreeningÂ
Goals and Types of ProcessingÂ
Fama offers a Software-as-a-Solution that helps companies identify workplace misconduct in the talent screening and due diligence sector. The solution uses a combination of AI and trained, human analysts to uncover evidence of workplace misconduct where an individual has provided such evidence via publicly available websites. Fama does not score, give a thumbs up or down, or offer a judgment about a person. Instead, Fama enables an enterprise client to define what workplace misconduct looks like to them online, reflecting their business purpose and need. Famaâs software, combined with a human analyst, will find a personâs web presence and add a source (referring to a candidateâs social media profiles, web content, or news articles) to the report only if it matches at least 3 identifiers about the subject. The solution then uses AI to read text and images just like a person can, highlighting examples on the subjectâs web presence that relate to one of the customerâs workplace misconduct criteria.Â
Fama also always allows Data Subjects the opportunity to request a copy of their report, request to be âforgotten,â and challenge the results appropriately.
The Nature of Fama Data ProcessingÂ
To initiate its check, Fama uses personal data such as a data subjectâs name, date of birth, email address, location, employment, and education history to discover a personâs âfootprintâ on the public web (i.e. their âweb presenceâ). The source of this data is provided by the client and it is not shared with anyone.Â
Customers may define the data retention period, Fama defaults to retain subject data for the tenure of the customer agreement for auditing purposes, extended by any relevant statute of limitations.
Once Fama has identified a personâs web presence, matching the result against three identifiers provided by the client, the solution collects publicly available data such as social media posts, web results, and PDFs from online databases, to review and identify any potential signs of what the client identifies as workplace misconduct. The only content that the solution uses is the content that is publicly accessible with an internet connection.Â
The risk profile of this processing relates to the automated collecting of the data subjectâs personal information. Note that at this point in time, the decision making hasnât occurred. This processing is merely aggregating all the verifiable, publicly available data on the web.â
The Scope of ProcessingÂ
Fama only collects this data once about a data subject, unless explicitly directed by a user in cases of ârescreeningâ and uses it only once to initiate the check about the data subject. The data will be kept for the lifetime of the agreement of the business customer by default, or deleted on a faster schedule depending upon customer preference.Â
Fama screens tens of thousands of people per month globally.
The Context of ProcessingÂ
Fama has a direct relationship with the data subject as a screening provider, hired on behalf of the company that is performing that screening. Data subjects engage with Fama directly to request copies of their reports, challenge results, or to be removed from our database. Given that these individuals are engaging with their future employer/investor in a pre-employment or pre-investment context, these individuals are aware of what Fama is doing on the companyâs behalf. Many of Famaâs clients collect consent prior to Famaâs interaction with the data subject in order to perform these checks; further making the interplay between parties explicit. â¨
Fama does not screen anyone under the age of 18 without proper parental or guardian consent.â¨
The use of algorithmic decision making has already been the subject of prior concern related to this type of use within the broader category of background screening. As such, there has already been some level of evolution in the marketplace with regard to the tools used to demonstrate mitigation of risk. In the present case, Fama helps mitigate some of the concerns by pursuing best-in-class data and information security policies.Â
Fama offers a novel, AI-based software solution that it created to evolve the exclusively manual, human-intensive approach to this type of candidate screening. Historically, many business users would physically look at a personâs web presence for signs of workplace misconduct. This process is, in and of itself, potentially problematic under the GDPR as the process exposes companies to irrelevant information about data subjects, outside of the course of normal business. In other words, these HR or Investment Ops professionals would process personal information which was not relevant or proportional; potentially introducing bias. Consider a member of the hiring team might see that a person is disabled in a profile picture on social media â they could not âunseeâ that information and may use it in a hiring decision. Because disability status is not being reported on by Famaâs AI, this will not come up as a factor in Famaâs report.
Fama uses technology to âblindâ that same HR user to such irrelevant and disproportional information, and only escalates for user review information that actually matches a companyâs workplace misconduct criteria. Fama was the first to create this technology to reduce the likelihood of seeing irrelevant and disproportional information, pushing the industry forward. The company is part of the PBSA, a US-based background screening industry group. Prior to its deprecation, Fama was also Privacy Shield certified.
The Purpose for ProcessingÂ
Famaâs purpose is to help organizations hire and invest in great people. Organizations count on Fama to help identify the workplace misconduct that damages brands, negatively impacts workplace culture, and introduces the potential for violence and fraud.1 Given the obvious impact of missing relevant and proportional information as part of the pre-employment/pre-investment diligence process, many HR or investment professionals are doing these sorts of checks manually.2 People manually searching the internet for insights about a candidateâs history of misconduct introduces the potential for bias and unintended consequences to the data subject being screened. Working with Fama alleviates and reduces that exposure while also providing the data needed to avoid misconduct. As such, Famaâs solution is designed to actually protect the fundamental rights and freedoms under the GDPR as it relates to the appropriate use limitation principles found in GDPR Article 5(1).
Famaâs Engagement with StakeholdersÂ
Fama maintains a standard cadence for updating and iterating its platform and solutions. In 2023, Fama launched new iterations to its software every two weeks, with a wide array of inputs from individuals across the organization. In addition to tapping teams such as sales, marketing, operations, HR and finance to influence the roadmap and approach to technology, Fama partners with a wide range of external constituents to create great customer experiences. For example, it uses law firms such as Seyfarth Shaw, LLP to craft its approach to FCRA and privacy compliance; embedding the firmâs guidance into the solution itself. Given that Fama operates in the highly regulated world of employment and investments, the approach has been to lead with compliance to help ensure clients and data subjects are protected using the solution. Whether it is the CTO writing about our approach to building ethical AI,3 or consulting with international development groups and advisors to scale products, Fama considers the approach to development/building as dynamic and always made better by external inputs.
Compliance and ProportionalityÂ
The lawful basis that Fama has for processing relates to its approach to ensuring maximum possible accuracy in reporting, only highlighting information on a report for which there is a business purpose to view, giving data subjects the right to view, contest, or delete their content from our systems, and providing the technical foundation to enable a business customer to define what âbusiness purposeâ is in their own context. Also, as noted earlier, the solution supports the principle of relevance and proportionality with regard to what personal data is used for the particular purpose. The technical âweeding outâ of irrelevant and disproportional data is one of the core features of the solution.
We safeguard all of our data, and customer and individual data, within our dedicated cloud server solution. Fama maintains an unqualified SOC II assessment, demonstrating an industry recognized compliance posture in data protection and security. This means that Fama both safeguards data and ensures that our processors comply with those safeguards, but also is functionally committed to data subjectsâ fundamental rights and freedoms as reflected in the GDPR.
âConclusionÂ
Today, we sit at the intersection of rapidly evolving technology and regulation. The result is âprivacy by design and defaultâ is even more critical in order to protect the individual rights and freedoms of individuals. This is a core principle of what has been coined as âethical AI.â Â
The nascent stage of AI and algorithmic tools in hiring requires a cautious approach. The field is evolving dynamically, with legal and ethical frameworks struggling to keep pace with technological advancements. In this landscape, leaders will emerge due to a responsible and innovative use of AI , striking a delicate balance between technological efficiency, human intervention and ethical responsibility.
AI and algorithmic decision-making are not merely tools; they are extensions of our collective ambition to foster a workplace that is efficient, fair, and respectful of individual rights and dignity. In this endeavor, Famaâs journey is illustrative of the broader trajectory that companies in this space need to adopt. Itâs a path focused on continuous learning, adaptation, and a commitment to building solutions that are not only technologically advanced but also humanely responsible and legally sound.
In conclusion, the future, with all of its promise and potential, awaits those ready to approach it with a clear vision, a robust understanding of the legal landscape, and an unyielding commitment to ethical principles and data subject rights.Â
âAbout John Tomaszewski, Esq.Â
John P. Tomaszewski is a leading Partner at Seyfarth Shaq LLP, with a focus on Privacy & Cybersecurity. John focuces on emerging technology and its application to business. His primary focus has been developing ttrust models to enable new and discuptive technologies and businesses to thrive. In the "Information Age", management needs to have good and counsel on how to protect the capital asset which heretofore has been left to the IT specialists-its data.
âAbout Seyfarth Shaw LLP
At Seyfarth, we are leading the way in delivering legal services more effectively, more efficiently, and more transparently. With more than 900 lawyers across 18 offices, Seyfarth provides advisory, litigation, and transactional legal services to clients worldwide. Our high-caliber legal representation and advanced delivery capabilities allow us to take on our clientsâ unique challenges and opportunitiesâno matter the scale or complexity. Our drive for excellence leads us to seek out better ways to work with our clients and each other. We have been first-to-market on many legal service delivery innovationsâand we continue to break new ground with our clients every day.
Seyfarth is a leader in global privacy and data security. We have considerable experience in multijurisdictional audits, as well as creating and implementing strategic international privacy compliance programs. We regularly advise multinational clients with respect to the collection, use and cross-border transfer of personally identifiable information of employees, service providers/vendors and other individuals, in order to comply with laws globally.